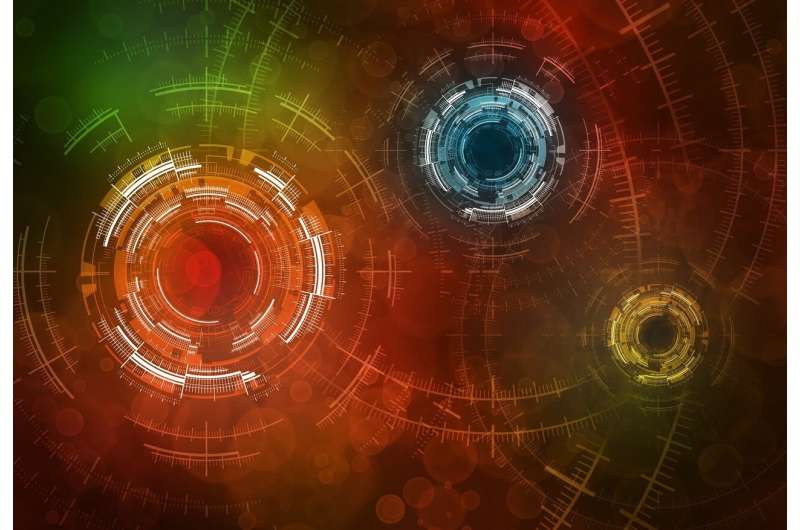
Irrespective of their personal, professional and social circumstances, different individuals can experience varying levels of life satisfaction, fulfillment and happiness. This general measure of life satisfaction, broadly referred to as “well-being,” has been the key focus of numerous psychological studies.
Better understanding the many factors contributing to well-being could help to devise personalized and targeted interventions aimed at improving people’s levels of fulfillment. While many past studies have tried to delineate these factors, few have done so leveraging the advanced machine learning models available today.
Machine learning models are designed to analyze large amounts of data, unveiling hidden patterns and making accurate predictions. Using these tools to analyze data collected in previous studies in neuroscience and psychology could help to shed light on the environmental and genetic factors influencing well-being.
Researchers at Vrije Universiteit Amsterdam and Amsterdam University Medical Center recently set out to investigate what predicts well-being by analyzing a large dataset collected in the Netherlands over a ten-year period using machine learning. Their findings, published in Nature Mental Health, pinpoint several factors that could predict well-being in the general population.
“Effective personalized well-being interventions require the ability to predict who will thrive or not, and the understanding of underlying mechanisms,” Dirk H. M. Pelt, Philippe C. Habets and their colleagues wrote in their paper.
“Using longitudinal data of a large population cohort (the Netherlands Twin Register, collected 1991–2022), we aim to build machine learning prediction models for adult well-being from the exposome and genome, and identify the most predictive factors (N between 702 and 5874).”
Pelt, Habets and their colleagues used machine learning models to analyze the Netherlands Twin Register, a dataset collected from a large population cohort over the course of 11 years. This data was collected from the same children when they were around 3, 5, 7, 10, 12, 14 and 15 years old, as well as from three distinct waves of adult participants.

The Netherland Twin Register dataset includes genetic information known as polygenic scores (i.e., genome), as well as information about the participants’ environment (i.e., general exposome) and psychosocial conditions (i.e., specific exposome). The researchers trained three different machine learning models, dubbed XGBoost (XGB), SVM and RF on this large amount of data.
They then used a powerful technique known as Shapley Additive Explanation (SHAP) to explore the contributions of different features to the predictions made by the three models. Their analysis revealed that the model’s predictions of well-being were based on various environmental and psychosocial factors related to the participants’ life satisfaction, happiness and quality of life.
“The specific exposome was captured by parent and self-reports of psychosocial factors from childhood to adulthood, the genome was described by polygenic scores, and the general exposome was captured by linkage of participants’ postal codes to objective, registry-based exposures,” wrote Pelt, Habets and their colleagues.
“Not the genome (R2 = −0.007 [−0.026–0.010]), but the general exposome (R2 = 0.047 [0.015–0.076]) and especially the specific exposome (R2 = 0.702 [0.637–0.753]) were predictive of well-being in an independent test set. Adding the genome (P = 0.334) and general exposome (P = 0.695) independently or jointly (P = 0.029) beyond the specific exposome did not improve prediction.”
Overall, the researchers observed that the genetic predispositions of participants (i.e., their genome) did not predict their reported well-being, while environmental and psychosocial factors did. The factors that they found to be most predictive of well-being are optimism, personality traits, social support, neighborhood dynamics and housing characteristics.
“Our findings highlight the importance of longitudinal monitoring and promises of different data modalities for well-being prediction,” wrote the researchers.
This recent study by Pelt, Habets and his colleagues suggests that specific environmental, social and psychological circumstances most contribute to people’s subjective well-being. In the future, the findings it gathered could inform the development of personalized interventions aimed at improving the life satisfaction of specific individuals, while also potentially inspiring the further use of machine learning to explore the factors influencing well-being.
More information:
Dirk H. M. Pelt et al, Building machine learning prediction models for well-being using predictors from the exposome and genome in a population cohort, Nature Mental Health (2024). DOI: 10.1038/s44220-024-00294-2
© 2024 Science X Network
Citation:
Using machine learning to uncover predictors of well-being (2024, September 13)
retrieved 13 September 2024
from https://medicalxpress.com/news/2024-09-machine-uncover-predictors.html
This document is subject to copyright. Apart from any fair dealing for the purpose of private study or research, no
part may be reproduced without the written permission. The content is provided for information purposes only.